Introduction: Reconfiguring the Landscape of Synthetic Media
The advent of OpenAIβs Sora represents a foundational breakthrough in the rapidly advancing trajectory of artificial intelligence, particularly in the sphere of generative media synthesis. This large-scale, multimodal model demonstrates an unparalleled capacity to produce high-resolution, temporally coherent video sequences directly from natural language prompts. Sora marks a decisive evolution from prior generative models by not only internalizing spatial and visual composition but also capturing complex temporal dynamics and contextual interrelations across frames. Since its limited release in early 2024, Sora has ignited intense discussion across multiple domainsβincluding media production, education, scientific visualization, policy communication, and ethicsβhighlighting its transformative potential.
Soraβs capabilities challenge traditional boundaries in digital storytelling, visual simulation fidelity, and the epistemological status of synthetic content. We are entering an era in which artificial intelligence can autonomously construct extended visual narratives that exhibit internal cinematic logic, material realism, and semantic coherence. Such a development necessitates a deep reassessment of how media is produced, interpreted, and trusted. This extended analysis explores Soraβs technical architecture, core mechanisms, cross-sector applications, systemic constraints, and broader philosophical implications in relation to existing AI ecosystems.
Model Overview: From Prompt to Temporally Stable Cinematic Output
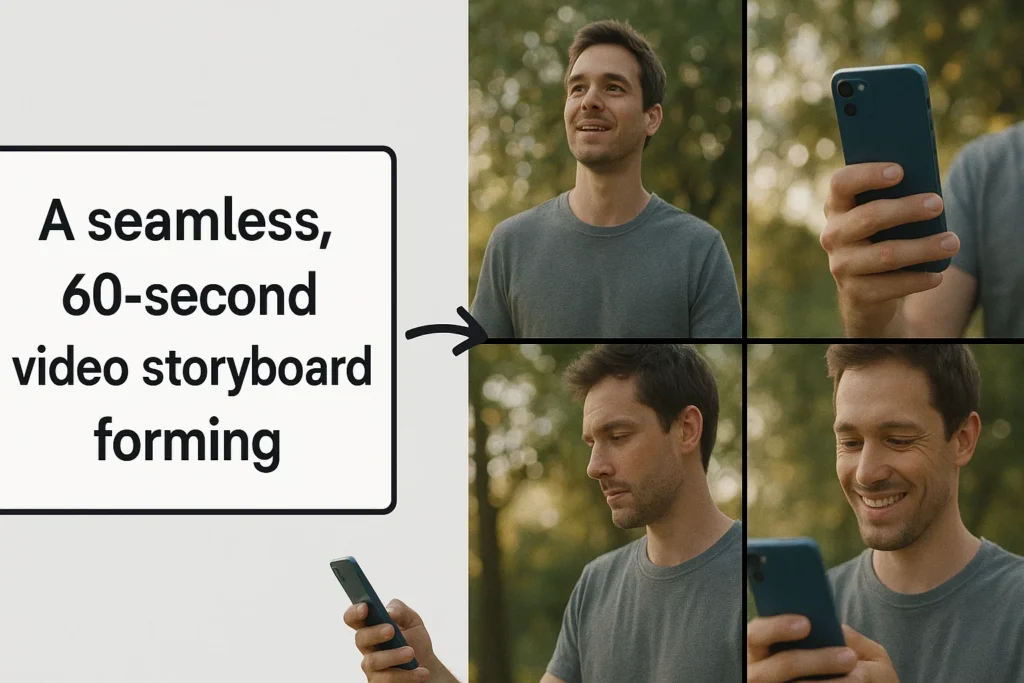
Sora represents OpenAIβs premier entry into high-fidelity text-to-video generation through the implementation of cutting-edge diffusion-based learning systems. It sets a new benchmark by enabling the generation of videos extending up to one minute in durationβfar exceeding the temporal capacity of any prior modelβwithout sacrificing visual consistency, realism, or semantic logic across frames.
The modelβs distinction lies in its ability to generate temporally continuous and visually coherent sequences that not only sustain a consistent aesthetic but also respect plausible physical laws and object behaviors. For example, Sora can accurately track the motion of dynamic agentsβsuch as a cyclist turning a corner or a glass shattering on impactβwhile preserving contextual continuity and spatial relationships. This makes it highly relevant for domains requiring embodied cognition, fluid narrative progression, and dynamic spatial reasoning.
Architectural Foundations and Functional Mechanics
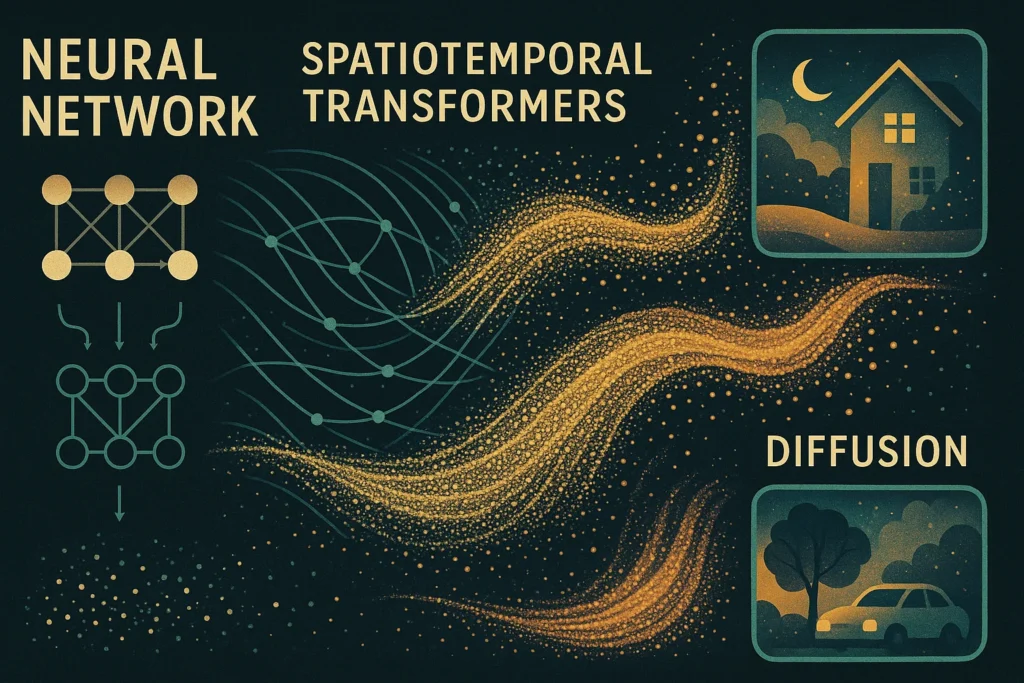
Although the precise specifications of Soraβs internal architecture remain proprietary, OpenAI has disclosed several innovative design features that collectively account for its superior generative capabilities:
- Diffusion-Based Synthesis: Based on the denoising diffusion probabilistic model (DDPM) paradigm, Sora iteratively refines noisy video sequences over time, enabling the preservation of fine-grained detail and dynamic motion.
- Spatiotemporal Transformer Modules: These enhanced transformer layers allow for frame-to-frame semantic attention, enabling temporally fluid transitions and cross-frame dependencies critical for narrative cohesion.
- Hierarchical Prompt Parsing: In order to direct item placement, scene transitions, and narrative arcs, complex textual inputs are broken down into layered interpretative components.
- Multimodal and Multidomain Training Data: By being exposed to an extensive dataset comprising cinematic works, scientific simulations, animated sequences, and naturalistic footage, Sora has learned to emulate diverse styles, genres, and temporal patterns.
- Scene Memory and Temporal Windows: The model appears to utilize internal mechanisms for retaining and reintegrating past visual states, thereby supporting long-range temporal coherence and reentrant object continuity.
Together, these architectural advances afford Sora a simulation-like quality, wherein its outputs exhibit emergent behavior indicative of a sophisticated understanding of physical causality, human movement patterns, narrative structure, and artistic framing.
Demonstrative Capabilities and Initial Reception
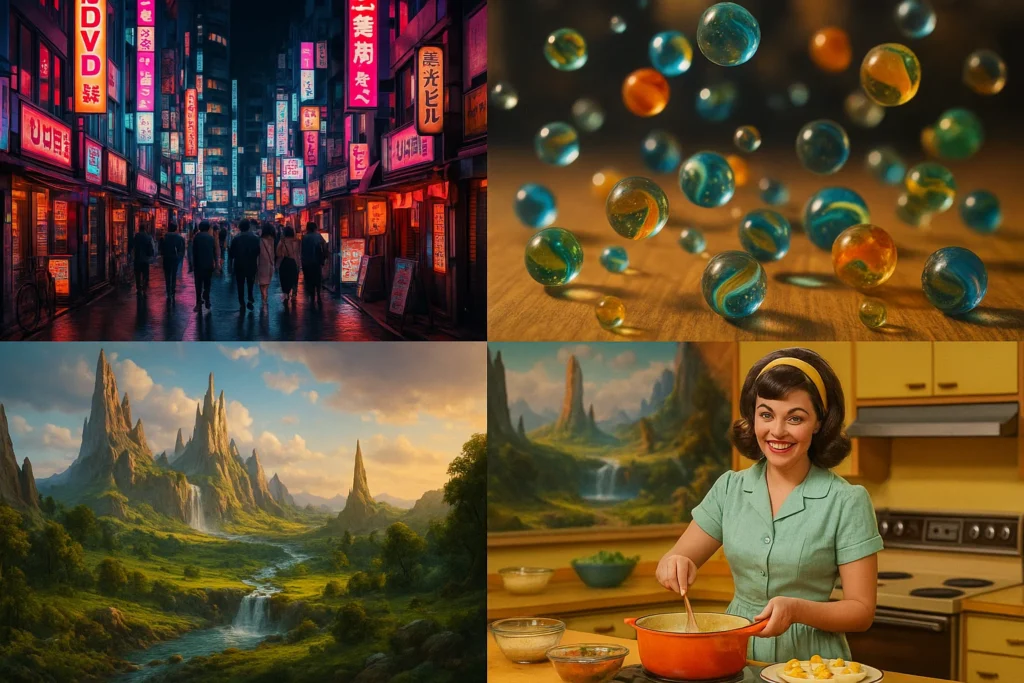
When it was first released, OpenAI displayed a carefully chosen collection of sample videos that demonstrated Sora’s wide range of creative abilities:
- A pedestrian’s trek through a snow-covered Tokyo at night, depicted with cinematic perspective and realistic ambient lighting.
- Glass marbles cascading and shattering in high-definition slow motion, exemplifying precise physics modeling.
- A drone-like aerial shot over a surreal fantasy landscape with morphing geological features and bioluminescent flora.
- A 1970s-style cooking tutorial with fluid camera transitions and detailed texture rendering of ingredients.
These demonstrations captivated audiences across technical and creative sectors. AI researchers praised its model stability and motion coherence, while artists and filmmakers explored its capacity as a rapid ideation tool. Educators expressed excitement over its potential to reimagine visual pedagogy, especially in disciplines requiring vivid procedural illustration. Collectively, these early reactions established Sora as a landmark achievement in synthetic audiovisual narrative.
Sectoral Impact: From Experimentation to Integration

The potential disruptions and innovations enabled by Sora extend well beyond speculative interest and into practical, cross-sector adoption:
- Cinematic Production and Indie Filmmaking: Directors and screenwriters can rapidly iterate visual concepts, storyboard sequences, and even produce polished short films with limited technical infrastructure.
- Marketing, Branding, and Content Personalization: Firms can develop hyper-targeted campaigns with demographically specific themes, visuals, and styles, eliminating logistical bottlenecks associated with traditional video production.
- STEM and Interdisciplinary Education: Educators can create interactive, context-rich visualizations of abstract or complex topics, enhancing learning outcomes across sciences, humanities, and arts.
- Game Development and XR Simulation: Designers gain the ability to simulate environments, pre-visualize interactive systems, and test real-time reactions in virtual settings without extensive 3D rendering pipelines.
- Public Sector and Civic Engagement: Municipal agencies can prototype urban development scenarios, visualize infrastructure projects, and simulate policy outcomes with public-facing narratives.
- Cultural Preservation and Media Access: Indigenous languages and oral histories can be revitalized and documented through visual storytelling powered by generative AI, fostering digital heritage preservation.
Sora’s adaptability and generative scope represent a powerful new layer in digital content pipelines, enabling novel workflows and empowering creators across a range of disciplines.
Technical Constraints and Ethical Considerations
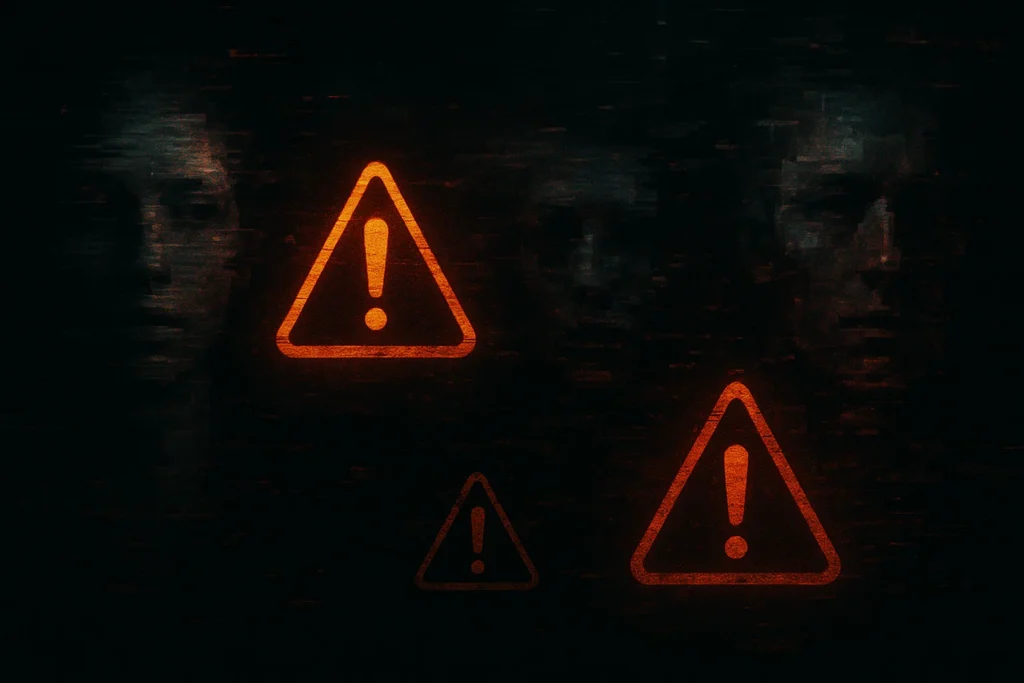
Despite its substantial achievements, Sora remains subject to several technical and ethical constraints:
- Temporal Anomalies and Visual Artifacts: In sequences involving complex interactions or intricate motion paths, the model may produce distorted limbs, inconsistent object shapes, or continuity gaps.
- Misinterpretation of Abstract Language: Prompts involving metaphorical, ironic, or symbolically dense language often result in literal or visually incoherent interpretations.
- Training Data Biases: The modelβs outputs may reflect prevailing cultural aesthetics and stereotypes, potentially marginalizing underrepresented narratives and identities.
- Risks of Misuse: The realism of Sora-generated video content introduces serious risks related to misinformation, synthetic identity creation, political manipulation, and non-consensual media generation.
To mitigate these risks, OpenAI has employed a multi-tiered safety framework involving internal red-teaming, staged deployment, visual watermarking, content moderation systems, and collaboration with stakeholders from policy, ethics, and civil society sectors.
Benchmarking Sora: Comparative Evaluation in the Generative Ecosystem
It is crucial to compare OpenAI’s Sora’s performance, adaptability, and coherence against those of current generative video systems in order to completely understand its disruptive potential. While a tabular comparison offers clarity, a deeper qualitative and strategic evaluation reveals how Sora positions itself as a paradigm-shifting innovation within the generative AI hierarchy.
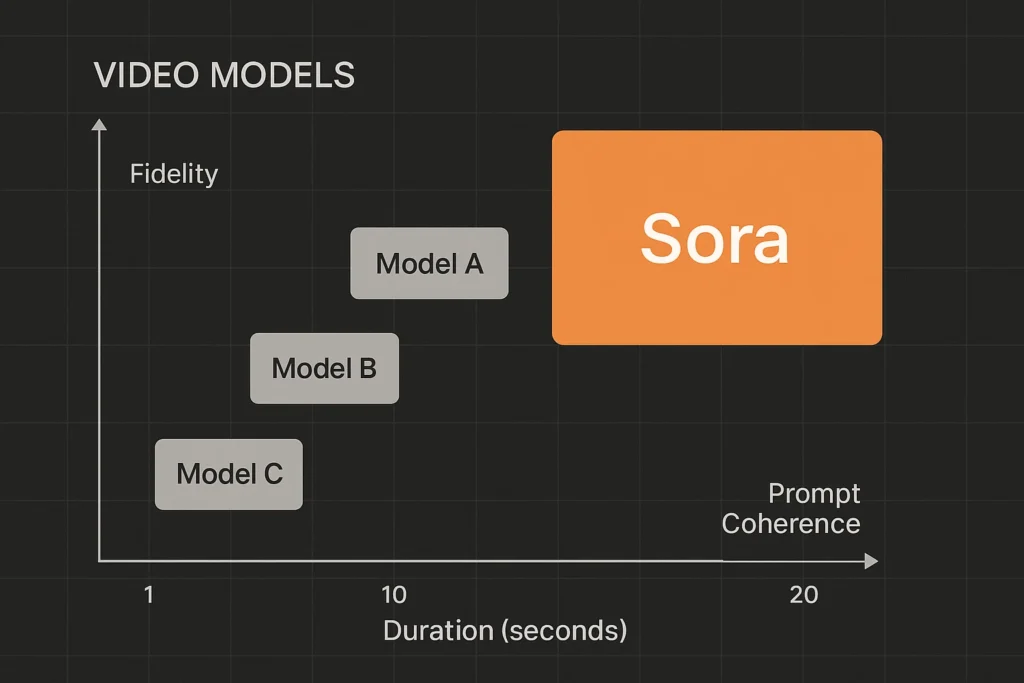
Temporal Superiority and Narrative Continuity
Soraβs ability to generate video content up to 60 seconds in lengthβwith consistent visual continuity, object permanence, and logical progressionβmarks a dramatic leap over existing models such as Runway Gen-2, Google Imagen Video, and Metaβs Make-A-Video, which typically plateau at around 4β5 seconds. This temporal extension is not merely quantitative; it enables the modeling of scenes with cinematic rhythm, causal sequencing, and character interaction. Such coherence is vital for narrative-based applications in filmmaking, simulation, and pedagogy, where longer arcs are required to convey complex scenarios or didactic sequences.
Semantic Precision and Prompt Alignment
One of Soraβs defining strengths is its high prompt sensitivity and interpretive depth. While many existing systems struggle with accurately capturing nuanced instructionsβparticularly when prompts contain nested clauses, abstract concepts, or temporal modifiersβSora demonstrates a significantly higher capacity for semantic parsing and contextual fidelity. This responsiveness is rooted in its hierarchical prompt decomposition architecture, which translates linguistic prompts into structured generative directives governing scene construction, motion trajectories, and object behaviors.
Multimodal Depth and Genre Fluidity
Unlike its predecessors, which often display stylistic rigidity or limited thematic scope, Sora exhibits remarkable genre fluidity and multimodal fluency. It is capable of producing outputs ranging from photorealistic cityscapes and stylized animations to surreal dreamscapes and scientific simulations. This versatility is attributed to its training on an unusually wide spectrum of multimodal datasets, encompassing film archives, game environments, procedural simulations, and synthetic imagery. As a result, Sora can shift across narrative registers and artistic traditions with minimal user intervention.
Spatial-Temporal Learning and Physical Consistency
Soraβs superior temporal coherence is coupled with an unprecedented level of physical realism. It consistently models accurate trajectories, collisions, lighting dynamics, and environmental interactions that mirror the laws of physics. Competing systems often falter in this domain, producing visually plausible but physically implausible scenesβsuch as floating objects, jerky transitions, or inconsistent shadows. Sora, by contrast, appears to incorporate spatiotemporal priors that inform not only motion but also material behavior, surface interaction, and volumetric consistency.
Ecosystem Integration and Systemic Positioning
From a systems integration perspective, Sora is being gradually positioned as a keystone within OpenAIβs broader suite of multimodal toolsβalongside ChatGPT, Whisper, and DALLΒ·E. Its architecture is already designed to interface with language models and third-party creative tools, pointing toward a future where multimodal creativity unfolds across interconnected layers of generative systems. In contrast, many competing models remain siloed or offer limited interoperability, reducing their applicability in professional production pipelines.
Benchmarked Performance Metrics and Implications
The tabular comparison below encapsulates Soraβs edge across six critical dimensions:
Feature | Sora | Runway Gen-2 | Google Imagen Video | Meta Make-A-Video |
Max Video Length | 60 seconds | ~4 seconds | ~5 seconds | ~5 seconds |
Resolution | HD+ | Medium | Medium | Medium |
Scene Complexity | High | Moderate | Moderate | Low |
Temporal Coherence | Strong | Moderate | Weak | Weak |
Prompt Sensitivity | High | Moderate | Low | Low |
Genre Variability | Extensive | Moderate | Limited | Limited |
These metrics are not merely comparativeβthey are indicative of a redefinition in the performance ceiling for generative video AI. Sora’s holistic advantages across resolution, duration, semantic richness, and contextual fidelity place it several iterations ahead of its peers.
Strategic Differentiation and Future Trajectory
What emerges from this evaluation is not only a technical superiority but also a strategic shift in design philosophy. Where other models have focused on modular improvementsβe.g., higher frame rates or better texture generationβSora reflects a more integrated ambition: to construct a platform capable of modeling narrative, perception, and interaction at scale. This sets the stage for future expansions into interactive video synthesis, real-time mixed-reality applications, and AI-augmented cinematic production.
Access, Deployment, and Future Roadmap
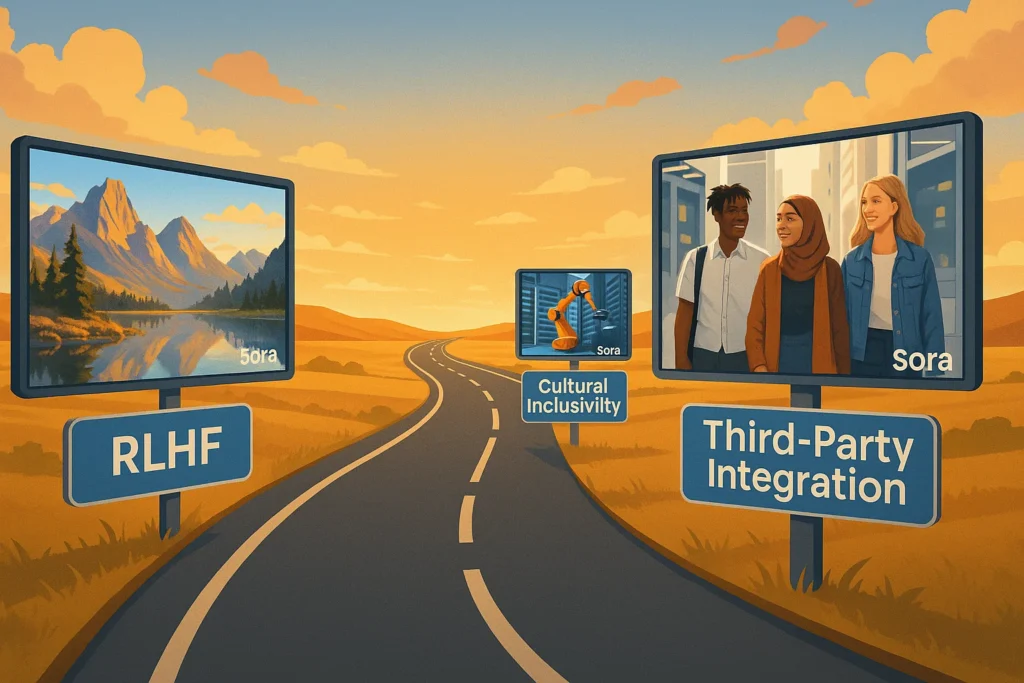
As of Q2 2025, Sora remains in restricted release, with access limited to selected collaborators in journalism, scientific communication, digital arts, and governmental use cases. OpenAI has committed to a deliberate rollout strategy, governed by ongoing research, community feedback, and safety enhancements.
Future development objectives include:
- Expanding linguistic and cultural prompt support to ensure global inclusivity.
- Smooth interaction with third-party creative tools and the ChatGPT interface.
- Enhancing alignment and factuality through reinforcement learning from human feedback (RLHF).
- Launching open research platforms to study emergent behaviors and develop shared ethical frameworks.
These efforts reflect OpenAIβs commitment to collaborative governance and sustainable AI integration, rather than unchecked commercialization.
Philosophical and Epistemological Reverberations
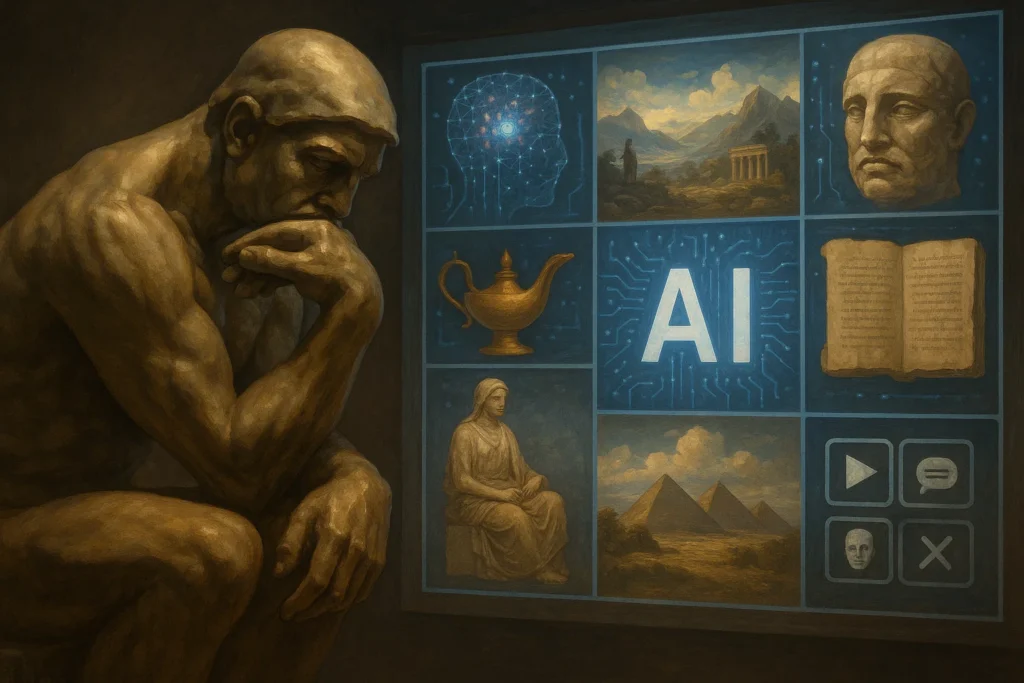
Soraβs advent forces us to confront fundamental questions about authorship, creativity, authenticity, and the epistemology of visual media:
- What constitutes authorship when content emerges from a hybrid of human intention and machine inference?
- How should we treat AI-generated visuals as documentary evidence in cultural or scientific contexts?
- Can synthetic memory serve as a legitimate conduit for preserving human heritage and collective experience?
- What new interpretive literacies are needed to decode AI-mediated narratives and distinguish synthetic from historical content?
These inquiries are increasingly animating discourse in the digital humanities, media studies, and cultural theory, casting Sora not merely as a tool but as a provocateur in reimagining the future of symbolic expression and mediated knowledge.
Conclusion: From Computational Innovation to Cultural Infrastructure
Sora exemplifies the shift from artificial intelligence as auxiliary novelty to AI as foundational infrastructure in the cultural, communicative, and cognitive domains. Its ability to generate plausible, layered, and expressive visual narratives from linguistic prompts signals the emergence of a new grammar of creationβone that fuses algorithmic generativity with human vision.
Rather than simply accelerating production, Sora redefines what is imaginable, rendering the intangible visible and expanding the bandwidth of expressive possibility. It invites a larger societal conversation around the ethical, aesthetic, and epistemic frameworks that should guide our integration of such powerful tools into public and private life. The challenge now is not only to build, but to govern, co-create, and critically reflect.